19th century handwritten documents are essential for researchers but are widely inaccessible even after digitization due to their inability to be searched. The Connecticut Digital Archive, a project of the UConn Library, is working to change that with a Catalyst Fund grant recently awarded by LYRASIS.
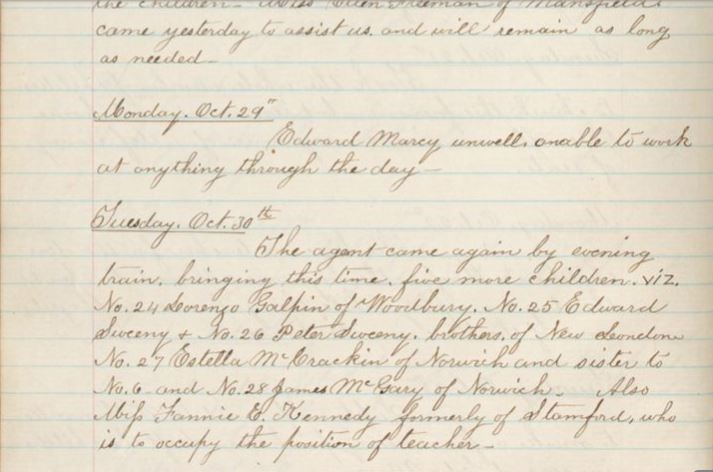
Archives and special collections from across Connecticut fill the Connecticut Digital Archive (CTDA), providing online access to a treasure of historic materials. However, even digitized, the irregularity in the handwriting in many of the manuscripts leaves the historical information in these documents inaccessible to Optical Character Recognition (OCR), a transfer method that has been used for more than 20 years to assist in document discoverability. To address this, historians and computer scientists have worked to apply machine learning to handwriting text recognition (HTR) through a relatively small number of projects with varied techniques and varied success.
In the summer of 2019, the Library, in partnership with Greenhouse Studios, the Massachusetts Historical Society, and UConn School of Engineering, created a set of over 16,000 images of 22 different characters from the John Quincy Adams Papers. These characters were used to train a neural network, or a set of algorithms modeled loosely after the human brain, designed to recognize patterns in those images. The neural network takes these handwritten digits, known as training examples, and develops a system to learn from them. As you increase the examples, the network learns more and improves its accuracy in identifying the individual letters and words. The pilot project over the summer produced promising results, with an 86%+ accuracy rate when testing on all 22 characters and an amazing 96%+ accuracy rate when testing on four of the characters.
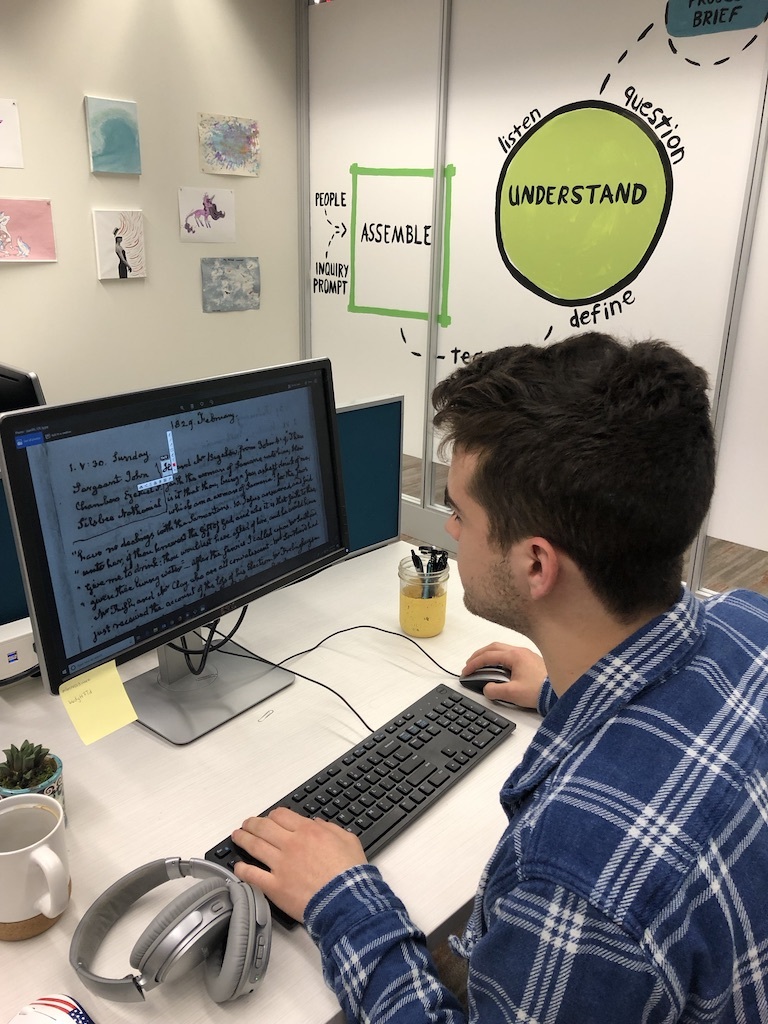
“Historical manuscripts are essential for humanities research and these funds will help scholars engage with unique and distinctive collections in a way they couldn’t before,” noted Greg Colati, Assistant University Librarian for University Archives, Special Collections & Digital Curation for the UConn Library.
The grant funds from LYRASIS will allow the Library and the Computer Science & Engineering Department in the School of Engineering to expand this work on additional volumes of handwritten documents in the John Adams Papers. The goal is to expand the datasets, adjust the neural networks, and release the updated version to the public for free.
LYRASIS is a non-profit organization whose mission is to support enduring access to the world’s shared academic, scientific and cultural heritage through leadership in open technologies, content services, digital solutions and collaboration with archives, libraries, museums and knowledge communities worldwide. The grant is part of their Catalyst Fund which provides support for new ideas and innovative projects that explore, test, refine and collaborate on innovations with community-wide impact.
The CTDA is a service of the UConn Library, providing services to preserve and make available digital assets related to Connecticut and created by Connecticut-based, not-for-profit educational, cultural, and historical institutions, including libraries, archives, galleries, and museums.